Artificial Intelligence (AI) has been constantly evolving and finding an emphatic presence across industries. The recent boom in cloud computing has played a pivotal role in the widespread adoption of AI.
AI-based data processing moved from on-premises to the cloud, and now, it has hit a new frontier – the Edge.
Businesses are now discovering the benefits of adopting Edge AI, i.e., AI-based data processing closer to the source where it is generated. Edge AI is basically a confluence of Edge Computing and Artificial Intelligence. It uses advanced analytics such as machine learning and edge computing techniques to make intelligent decisions in milliseconds!
Let us learn a little more about Edge AI in this blog.
How Does Edge AI Work?
Edge AI makes use of machine learning algorithms running directly at the edge. But what really is the edge? Let’s understand this by comparing edge computing with cloud computing.
We are all familiar with cloud computing, wherein information processing happens at a centralized server, i.e., the cloud server. Cloud computing is a disruptive technology because it facilitates enormous computing capabilities naturally. The devices connected to the cloud just transmit local data to the cloud, while retaining their key characteristics of low power consumption, minimal processing, and reduced cost.
In edge computing, the information is processed directly at field-deployed IoT devices, i.e., the edge. Edge computing is rapidly moving into the spotlight due to several reasons:
- Hardware for IoT edge devices has become more powerful, while remaining affordable.
- Optimization of software for edge devices and its benefits are widely acknowledged.
Leveraging the power of machine learning, IoT edge devices can now make intelligent decisions locally. They do not have to send the data collected to the cloud for analytics.
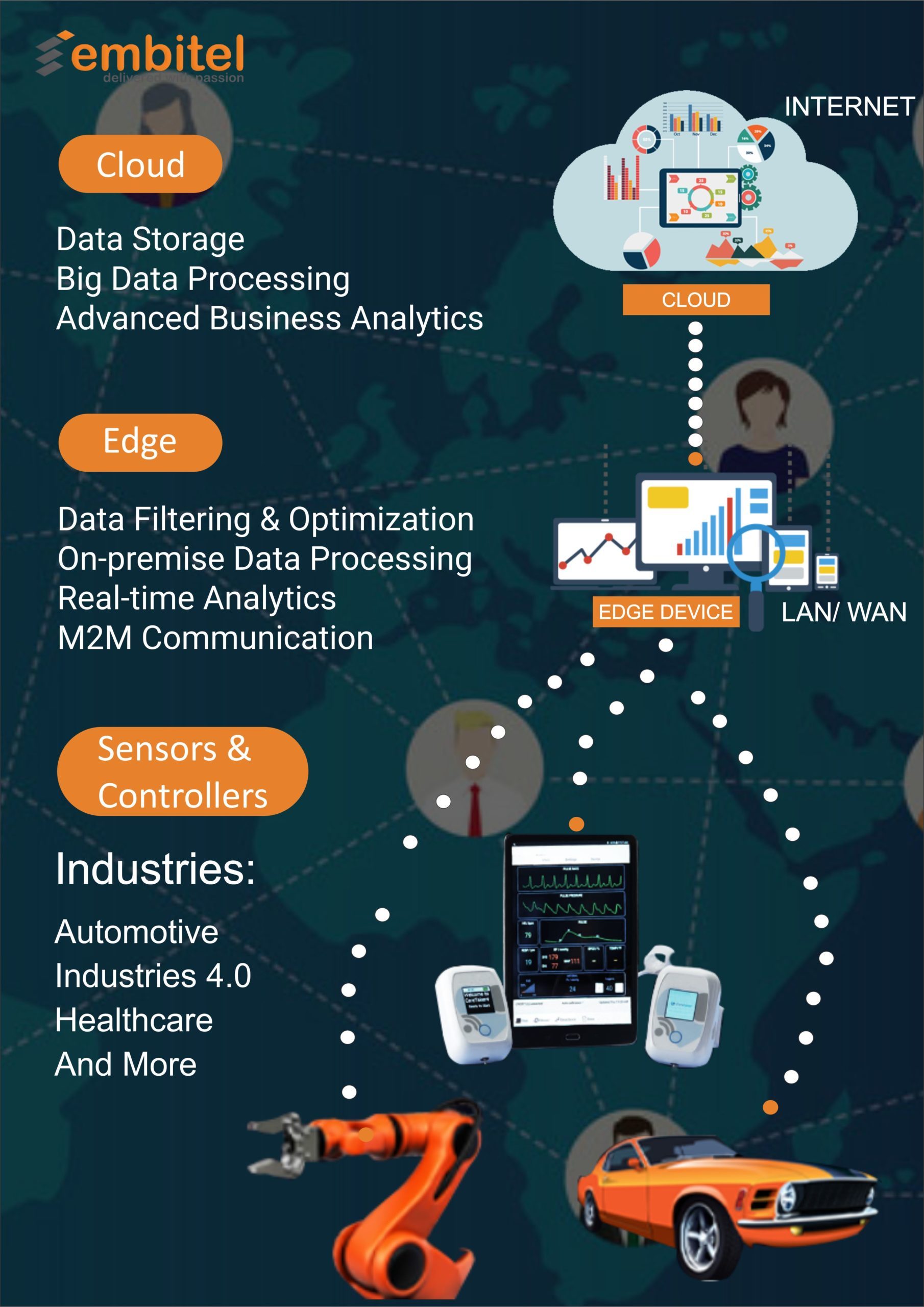
For instance, edge AI can be utilized for predictive maintenance of equipment in Industry 4.0. This implies that edge devices can analyze historical data to identify scenarios wherein an industrial machine may fail, and predict this before the actual failure happens.
With the development of machine learning, such complex processing and decision-making capabilities will be extended to IoT edge devices, across industries.
In order to facilitate Edge AI, each edge device needs to have an in-built microprocessor and sensors. The sensors help in collecting necessary data. The data processing is completed locally on the device and it can be stored locally as well. Later on, the edge device can connect to the internet and transmit this information to the cloud server for subsequent processing and storage. Since the edge device is not constantly connected to the cloud and exchanging data, it tends to have a longer battery life.
Will Edge Computing Replace Cloud Computing?
The simple answer to this question is NO. Edge computing is not expected to replace cloud computing. Instead, it will work in conjunction with cloud computing to leverage machine learning at scale.
It is well-known in the industry that cloud-based machine learning has great performance efficiency. Also, when implementing basic Edge AI, it becomes necessary to train the models in cloud-based environments as well. For some use cases, cloud computing can even be a better option.
Composite processing models are now being adopted by various organizations. In such models, only specific AI tasks (that demand low computing power) will be performed on IoT edge devices. Cloud computing will handle the deployment of complex machine learning models.
Edge Computing Growth Forecast
Edge computing technologies have restructured data computation and helped businesses derive valuable insights from data analytics in an accelerated manner.
A recent study by Million Insights indicates that the edge computing market was valued at USD 3.5 billion in 2019. It is expected to flourish at CAGR of 37% between 2020 and 2027.
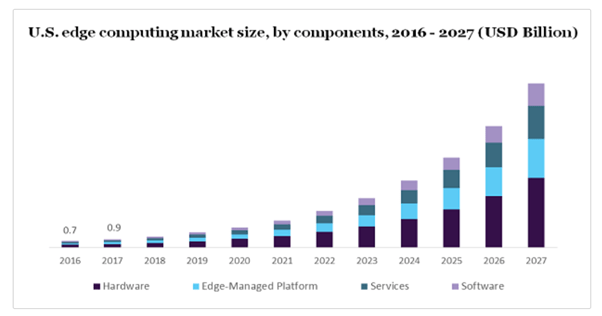
The report also suggests that edge computing is seeing increased adoption in the healthcare and manufacturing sectors post the Covid-19 pandemic. An example of Edge AI in healthcare is ensuring public safety through the use of drones and robots in hospitals. Edge AI generates real-time insights that help in identifying, isolating and treating Covid-19 patients at an accelerated pace.
7 Unbeatable Benefits of Edge AI
In Edge AI, since the data processing is done at the IoT edge devices, the entire process of decision-making speeds up. This also leads to better user experience. These are not the only advantages offered by Edge AI. Let’s explore the unsurpassable benefits offered by this technology in detail.
- Reduced Latency – With Edge AI, analytics is performed in milliseconds. This is possible because there is no need to send data to the cloud for processing. This reduces the latency in data processing and is crucial for use cases that are time-critical.
Let us take the example of predictive maintenance of industrial equipment again. If the ML algorithm on an edge device identifies that there is a possibility of equipment failure, then it can send a command to immediately shut down the equipment. On the other hand, if cloud computing was utilized for analytics in this scenario, there would be a delay of at least 100 milliseconds while the data is transmitted to and from the cloud to the device. In operation-critical equipment, this delay of a few seconds may result in a major breach of safety or quality!
- Scalability – There is tremendous growth in the number of interconnected IoT devices across industries. And Edge AI is an excellent way of ensuring efficient data processing, as it is not required to send all the data to the cloud. If there is a need to simultaneously process a large amount of data from thousands of sources in real-time, intelligent edge devices come to the rescue.
- Minimized Strain on Cloud – Edge AI reduces network strain by distributing the workload across multiple edge devices. Hence, the usage of CPU and memory in the cloud goes down.
- Information Security – As indicated above, with Edge AI the need to constantly send data to the cloud reduces. This also implies that the possibilities for security breaches and online attacks are lesser. When there is a network of IoT edge devices processing data, it is harder to bring such an ecosystem down than attacking a single data center/cloud server.
- Reduced Operational Risks – Since Edge AI follows a distributed model, the operational risks are spread across the network. This is a more reliable solution than depending on a centralized cloud server. In case the cloud system fails, the entire ecosystem will come to a standstill. However, in the case of Edge AI, the failure of a single edge device will not pose such a massive threat to the ecosystem.
- Cost Savings – Edge AI saves a lot of bandwidth, as the transfer of data is minimized. This also reduces the capacity requirements for cloud services. Hence, Edge AI is a cost-effective solution, when compared to cloud-based ML solutions.
- Democratization of AI – Edge AI is designed to be powered by affordable edge devices. Hence, AI now becomes accessible to organizations of various sizes and even to educational institutions.
Edge AI – Drawbacks that Cannot be Ignored
In several use cases, machine learning models are complex and often large in size. In such scenarios, it becomes impossible to shift these models to compact edge devices. If efforts are initiated to reduce the complexity or size of the algorithms, the accuracy of predictions will take a hit. In some situations, the IoT edge devices may also not be able to perform complex processing tasks, as their computing power is limited.
Hence, during the design phase of Edge AI devices, it is crucial to evaluate all these failure points. It is also essential to test the trained model exhaustively on different types of devices and various OS versions.
Organizations can start their Edge AI journey by initially applying this technology to applications that are not mission-critical. It is also important to identify the right edge location and cloud infrastructure in the initial phases.
High Performance Edge Computing
When engineers attempted to bring machine learning and deep learning to high-performance computers at the edge, the paradigm of High Performance Edge Computing (HPEC) was born. In this model, the high-performance computational capabilities (such as that of cloud computing) can be brought to sophisticated field-deployed edge computers.
The most crucial aspect in the implementation of high performance edge computing is the design of the edge device. While the device is compact, it should also be rugged and have supreme processing power. This necessitates the use of new classes of hardware, IP, and software on edge devices. The perfect balance of Operational Technology (OT) and Information Technology (IT) infrastructure is also critical for such solutions.
Hardware and Software Framework Suitable for Edge AI
Microcontrollers:
- Wio Terminal – With a powerful MCU and a highly integrated design, this Arduino development platform enables you to deploy an optimized machine learning model effortlessly.
- Seeeduino XIAO – This board is most suitable for TinyML due to its compact size.
Single-Board Computers (SBCs):
- ODYSSEY x86J4105800 – This product is one of the most powerful SBCs out there. It supports Linux and Windows 10 OS
- Coral Dev Board with 1GB RAM – This product is specifically designed for the development of neural network solutions using TensorFlow Lite.
- NVIDIA Jetson Nano 2GB Developer Kit – This budget-friendly option for Edge AI is powered by Quad-core ARM A57 CPU
In this context, it is also important to take a look at software frameworks that facilitate Edge AI. One such framework is EdgeX Foundry.
EdgeX Foundry is an open-source software framework that enables heterogeneous devices at the edge to communicate with each other and operate efficiently. This technology enables developers to quickly design edge applications by providing components to develop data collation, analytics, and command control. The services and layers in EdgeX Foundry are essentially a two-way transformation engine that connects cloud applications and IoT edge devices.
Noteworthy Use Cases of Edge AI
Striking applications of EdgeAI can be seen in the following domains:
- Autonomous Vehicles – In self-driving cars, Edge AI devices process data locally at great speed. In such a vehicle, sensors collect a huge amount of data in real-time and it needs to be processed immediately for the safe operation of the vehicle. For instance, the autonomous car needs to identify traffic signals instantly, analyze pedestrian movement and look out for hazards, all at the same time. Edge AI is pivotal in ensuring that the data processing occurs without any delay.
- Industrial IoT – Edge AI enables advanced industrial IoT assets such as robots to perform visual inspections of products on the production line and take appropriate decisions instantly. This also increases the efficiency of the plant.
- IoT in Healthcare – In the healthcare industry, Edge AI can facilitate the advanced monitoring and analysis of a patient’s health and predict early pathologies. Since the patient’s medical data is stored on-site, there is minimal risk of a security breach. This data can also be used to provide effective prescriptions to patients in real-time.
The industries that can benefit from Edge AI are many. While organizations are increasingly moving towards this decentralized computing architecture, they are also discovering new avenues where this technology can be implemented for improved efficiency.
Connect with us to discover how Edge AI can help your business conquer new frontiers through accelerated machine learning.