In the city of bright lights and sleek rides, a new era unfolded for automobile owners. The vehicles could recognize their owners at a glance and come alive with a futuristic hum. Keys were yesterday; cars in this city identified their owners like old friends with the help of face recognition technology.
In a world where automotive security troubles ranged from the annoying to the dangerous, this technology was a game-changer. No more fumbling for keys—security was as simple as looking at your car and feeling it respond. Not just that, integrating this feature enhances several existing features of the vehicle, helping to deliver a complete connected experience.
The growing trend and trust in intelligent solutions set a precedent for such technology to thrive! This article breaks down facial recognition in vehicular biometric technology, challenges to its integration and the wide range of applications in the connected vehicle realm.
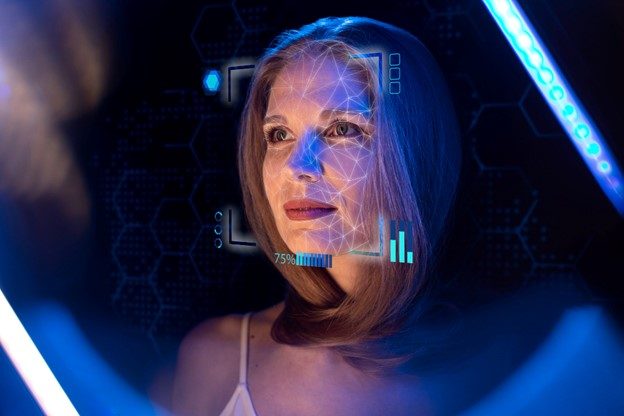
In the current scenario, Automotive OEMs are slowly, but surely, beginning to trust and integrate car face recognition as a reliable and modern security feature. Exhibit A - Hyundai's Genesis GV60.
The Need for Advanced Automotive Safety and Security
Technology has been evolving at lightning speed, but so are the threats associated with its secure usage. Automobile theft poses a real problem, with 1,001,967 vehicles reported stolen in the US during 2022 alone. Thus, integrating advanced security solutions to protect our prized assets on wheels is the need of the hour.
Implementing face recognition in cars not only helps the owners from preventing theft, but it can also ensure driver safety. With its inputs and tailor-made algorithms, the vehicle can assess the driver and offer necessary safety alerts and suggestions as well.
These alerts can be delivered through seat vibrations and alarms. Syncing the main infotainment unit and rear seat entertainment systems with car face recognition components can work wonders. It helps in effectively communicating alerts/notifications or conducting authentication processes to vehicle occupants.
The Origins
First things first, face recognition technology isn't new, but integrating it as a car safety feature sure is. Woodrow M Bledsoc and his team were the first to experiment with this futuristic solution between 1964 and 1966. The objective of the experiments was to look into the possibility of recognizing faces through computer programming.
However, due to the novelty at the time, the team faced several challenges to overcome the variability recognized by the computer. Appropriate solutions (algorithms) to nullify the challenges are suggested below:
Challenges reducing the efficiency of Face Recognition | Algorithms to nullify the challenges of Face Recognition |
---|---|
Head Rotation | Multi-Angle Detection |
Tilting | 3D Face Recognition |
Light intensity and Angles | Lighting or Illumination Normalization |
Facial Expression and Aging | Dynamic Feature Analysis and Independent Component Analysis |
Despite achieving accuracy up to 99.97%, some of these problems still haunt the accuracy of facial recognition.
Modern Facial Recognition systems utilize AI to quickly process the constant flow of data. However, Artificial Intelligence in Face Recognition is a maturing technology at the moment.
Various reports indicate that these systems manifest a higher error rate in identifying faces of color when compared to white faces. Hence, careful calibration phases need to be planned.
Recognizing Owners by their Facial Features - The Process
A conventional face recognition system typically entails the sequential processes of face detection, feature extraction, and processing.
Face recognition involves comparing an image against a stored repository of faces, aiming to ascertain the identity of the subject portrayed in the input image. Several pivotal factors, including shape, size, pose, occlusion, and illumination, intricately influence this identification process.
Primary face recognition focuses on discerning unique facial landmarks, including nose width, eye dimensions, jaw attributes, cheekbone elevation, and eye separation. This leads to the creation of a distinctive numerical code. Subsequently, the system undertakes a comparative analysis of this numerical code with another image, discerning the degree of similarity between the two pictures.
Automobiles such as cars follow a similar procedure to recognize faces!
Algorithms that enable Face Detection and Recognition in Automobiles
The development of AI & ML algorithms is the heart of face recognition technology in cars. They leverage advanced mathematical and computational techniques to extract meaningful features, learn patterns, and accurately predict facial recognition tasks.
While designing technology of this magnitude for automobiles, the following algorithms have resulted in highly accurate solutions:
Eigenface-based methods (PCA algorithm)
Principal Component Analysis (PCA) identifies patterns by transforming correlated variables (pixel values in face images) into a new set of uncorrelated variables called principal components. Eigenfaces are the principal components representing the most significant variations among face images.
The car uses these eigenfaces for recognizing and categorizing new faces, focusing on the features that contribute the most to the overall variability. Principal Component Analysis aims to decrease the complexity of the data by maintaining as much of the original dataset's variation as feasible.
Linear Discriminant Analysis (LDA)
LDA aims to find a linear combination of features that maximizes the separation between different classes (individual faces). It calculates the between-class (quantifies the variability of data points belonging to the same facial feature) and within-class (quantifies the separation between different facial features) scatter matrices to determine the optimal projection that maximizes differences between the faces of different people while minimizing variations within the same person's face.
This results in a set of features that effectively differentiates between individuals.
Independent Component Analysis (ICA)
ICA assumes that a face image is a linear combination of independent sources, each representing a specific facial feature. After breaking down the facial features into independent components, the algorithm isolates and identifies individual features. This robust approach allows the model to handle variations in lighting, expressions, and other factors.
Elastic Bunch Graph Matching Technique
This technique represents faces as interconnected nodes connected by elastic bands, forming a graph that captures the spatial relationships between facial features. The elasticity enables the model to adapt to variations in facial expressions or poses, providing a flexible and accurate representation to recognize faces in cars.
Neural networks (NN)
Neural networks consist of layers of interconnected nodes (neurons), each associated with weights that get adjusted during training. These networks learn hierarchical representations of features, capturing complex patterns in face images.
The activation of neurons in the output layer corresponds to the face classification, allowing the model to generalize well to new, unseen faces.
Support Vector Machine (SVM)
Support vector machines (SVM) classify faces by finding the hyperplane that maximally separates different face classes. Think of it like drawing a line between two groups of people in a room based on certain features, like height and hair color. The SVM is the brain deciding the best line (hyperplane) to differentiate the two groups.
So, when new faces come in, it can quickly assign the group they belong to based on their features. The system can create two groups, authorized and unauthorized to grant vehicle access! SVMs are particularly powerful when dealing with high-dimensional feature spaces.
Due to the increased emphasis on developing intelligent technologies, innovators in the automotive industry rely heavily on deep learning and artificial intelligence-based solutions.
Deep Learning and Artificial Intelligence for Face Recognition
Deep learning involves utilizing deep neural networks with multiple layers. It learns intricate features and representations from the dataset. Deep learning for facial recognition involves Convolutional Neural Networks (CNNs) or other specialized architectures.
CNNs excel at capturing spatial hierarchies, making them well-suited for image-based tasks like face recognition. Feature extraction and learning are the two other essential aspects of facial recognition, and these components are utilized in making cars safer.
a) Hierarchical Feature Extraction
- Deep networks learn features step by step.
- Early layers focus on basics like edges and textures.
- Deeper layers handle complex structures like facial contours.
b) Representation Learning
- Deep learning is best at finding meaningful patterns.
- By encoding faces into a space where similar faces are close, deep learning assists in identifying faces under different constraints.
- It can differentiate between individuals easily.
Through continuous learning and exposure to diverse examples, the AI based system's ability to recognize faces in cars will improve over time. Custom artificial intelligence and machine learning algorithms can help automotive OEMs to unlock a wide range of face recognition-based solutions.
Integrating Facial Recognition in Vehicles for Feature Enrichment
Face recognition technology offers a wide range of vehicle applications, significantly enhancing security, personalization, safety, and convenience. Understanding these applications provides insight into the opportunities and future of automotive technology to transform the driving experience.
Authentication
To authenticate drivers before allowing access to or starting the vehicle, this system uses pre-registered facial data to ensure that only authorized individuals can unlock and operate the car. The authentication process typically involves:
- Capturing the driver's facial image using an in-car camera.
- Comparing the captured image with stored data.
- Granting or denying access based on the match results.
In the event of an unauthorized user attempting to access the vehicle, face recognition technology can trigger alarms and send notifications to the owner and authorities.
By identifying passengers, the system can enable personalized settings or restrict access to specific vehicle functions.
An example of full-fledged usage of this technology is Hyundai's Genesis GV60. The IR Camera infrastructure is set up at the B-Pillar of the vehicle. This enables keyless authorization.
Personalized User Experience
Using face recognition, adjust various in-car settings to match the preferences of the recognized driver. This includes seat position, mirror angles, climate control settings, and more. The system uses the driver's profile to ensure a comfortable and personalized driving environment every time.
The technology can tailor the vehicle's infotainment system to the driver’s past preferences, including music playlists, radio stations, and navigation routes.
Futuristic features such as Advanced driver-assistance systems (ADAS) can also be adjusted based on the driver’s comfort level and experience.
Safety and Monitoring
Face recognition technology is integral to Driver Monitoring Systems, which track driver attentiveness by detecting signs of drowsiness, distraction, or intoxication. When the system identifies these signs, it can provide alerts or take corrective actions such as reducing vehicle speed or activating autonomous driving features.
The system can also detect signs of medical distress, such as sudden changes in the driver's facial expressions or posture. In such cases, it can alert emergency services, potentially saving lives by ensuring timely medical intervention.
By assessing the driver's emotional state, face recognition technology can recommend measures to improve their mood and concentration.
Fleet Management
Fleet operators can use face recognition to log driver identity, driving hours, and behavior. This data is crucial for compliance with regulations, as well as for analyzing and improving driver performance.
It also ensures that only authorized personnel can operate specific vehicles. This enhances security and prevents unauthorized use, which is particularly important for commercial fleets.
Convenience Features
Face recognition can facilitate seamless transactions for fuel, tolls, and parking. When the system recognizes the authorized driver, it can automatically process payments, saving time and enhancing convenience.
For security reasons, voice command systems can be restricted to recognized drivers only. This ensures that sensitive functions can only be activated by authorized individuals.
Face recognition technology can assist law enforcement by identifying known offenders or missing persons during routine patrols or traffic stops. This integration enhances public safety and aids in crime prevention.
Vehicles equipped with face recognition can link with public surveillance networks, providing valuable data for crime investigation and prevention. This integration helps create a more secure environment for all road users.
Conclusion
The journey towards widespread adoption of face recognition in cars is just the beginning. IoT solutions are the backbone, connecting the intricate network of sensors, cameras, and processing units. As face recognition technology evolves, the integration of IoT enables real-time communication and continuous improvement.
The synergy between face recognition and IoT empowers vehicles not only to recognize their owners but also to adapt and respond to dynamic safety challenges.
IoT service providers, such as Embitel, armed with expertise in AI and ML services, play a crucial role in shaping the future of automotive security. Our ability to develop and implement advanced algorithms will assist in harnessing the power of AI in the automotive context.